The Data Is Dark And Full Of Terrors: Advice for the Data Scientist Noob
Somebody asked me what the life of a data scientist is like. I’ve never liked the term, but I like the development in the IT business that…
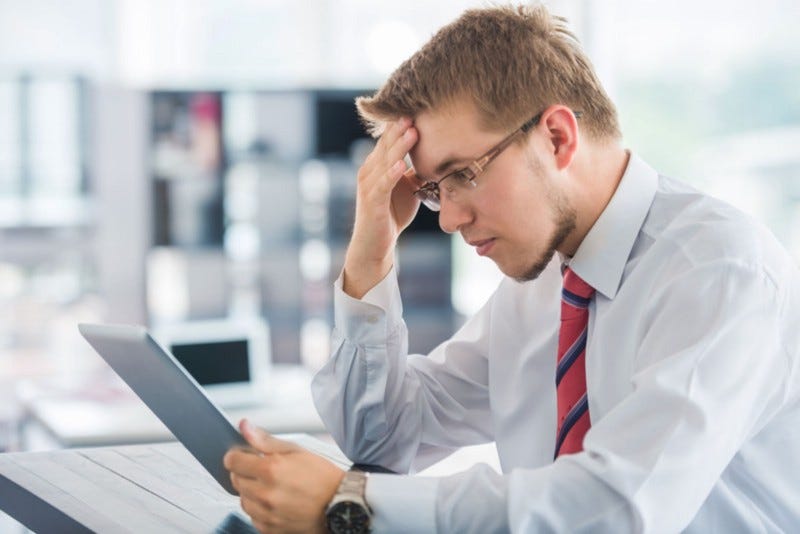
Somebody asked me what the life of a data scientist is like. I’ve never liked the term, but I like the development in the IT business that made the term necessary. So here’s my end all, be all answer.
I don’t think there’s a general purpose ‘data scientist’. I think, as I’ve always said, that such a person is someone who can code and knows statistics. A …
Keep reading with a 7-day free trial
Subscribe to Stoic Observations to keep reading this post and get 7 days of free access to the full post archives.